Addressing the Energy Crisis in a Biotech-Driven World by 2039
Explore innovative solutions to address the biotech industry's growing energy demands by 2039, focusing on hybrid energy systems, AI-driven optimization, efficient cooling, and quantum computing to ensure sustainability and minimize carbon footprint.
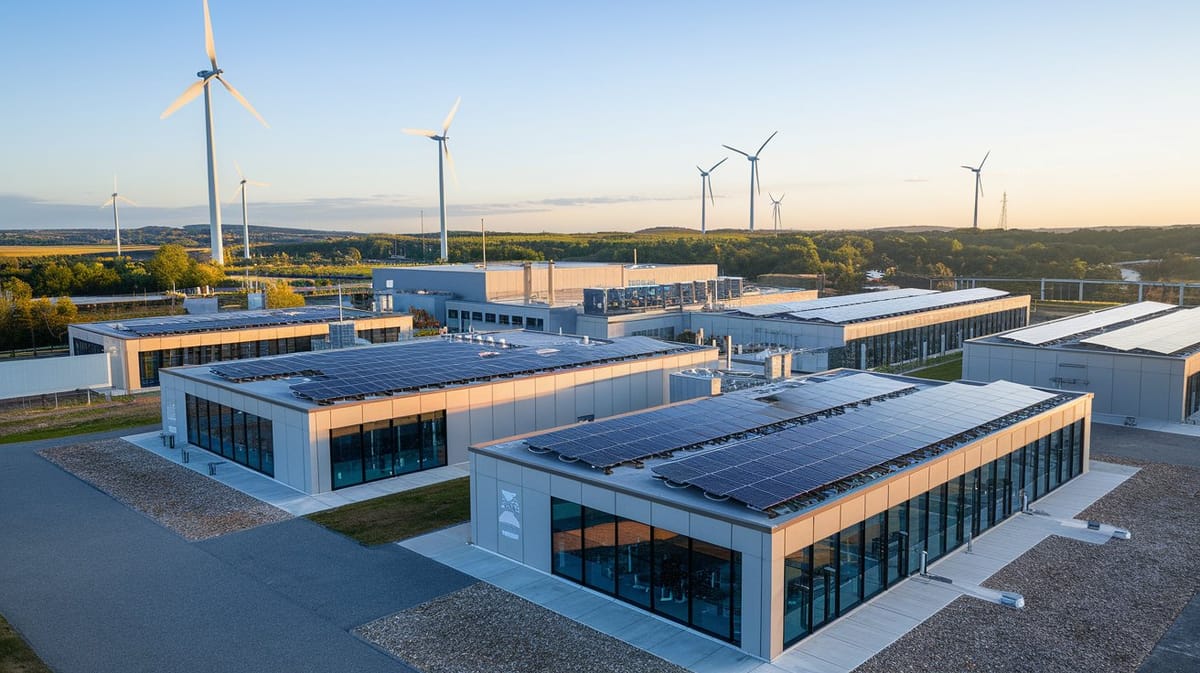
Global healthcare would have changed in ways unforeseen by 2039, with the biotech industry that has transformed into something of which individuals in this generation cannot even fathom. What has just been established is that AI-driven drug discovery, genomic sequencing, real-time health monitoring, and bioinformatics will evolve the methodology to revolutionize global healthcare. While these scientific discoveries continue to expand what is possible in medicine, demand for energy to power it has never been higher. The high energy requirements of AI models, the enormous generation of genomic data, and in-real-time analytics place tremendous pressure on the biotech sector-supported data centers. By 2039, expansions of infrastructure in the biotech sector will likely increase electricity requirements to 20-25% for data centers. This situation entails urgent, innovative solutions for the survival of the biotechnology industry not to be a subversive element against the global energy crisis.
The Energy Challenge in Biotech
The energy needs of the biotechnology industry are mainly dominated by high-performance computing and large-scale data storage. An illustration of this is AI-based drug development, which consumes thousands of times more energy than conventional methodologies, due to the complex modeling of biological systems and interactions. Also, genomic data are rapidly accumulating, and the number of data expected to be produced annually shall reach 100 zettabytes by 2039.
Key Challenges:
Energy Inefficiency in Biotech Data Centers: The vast amounts of data generated by genomic sequencing, personalized medicine, and biotech research are pushing data centers to their limits. Many rely on energy-inefficient cooling technologies and outdated infrastructure, unable to keep up with demand while minimizing their carbon footprint.
Energy Demand of AI and Quantum Computing in Biotech: The commercialization of quantum computing and AI in biotech—used for drug discovery, genome analysis, and bioinformatics—requires immense computational power, significantly increasing energy usage. Quantum computing, in particular, has high cooling and processing power requirements.
Environmental Impact of Biotech Infrastructure: The energy demands of the biotech industry are contributing to global warming and environmental degradation, with the carbon footprint of biotech-related data centers and devices accelerating climate change and natural resource depletion.
Solution to Energy Crisis
This crisis needs a multi-faceted approach on efficiency in energy, optimization in computing processes, and the integration of advanced technologies into biotech infrastructure. Below are intricate solutions directed at reducing consumption of energy while the growth of the biotech industry takes its right course:
Hybrid Energy Solutions
Hybrid energy system is one of the important solutions for the management of biotech data center demands. It integrates renewable source energy like that of the sun or wind with traditional grids. Integration of the renewable sources results in less reliance on fossil fuels as a substitute. The traditional energy grid, in this case, serves to act as a backup for the induction of fluctuating renewable source for ensuring a continuous power supply during a safe time.
In principle, such hybrid systems would level out energy consumption of data center's with the offers of energy supply whenever surpluses are produced surpassing demand and draw them from traditional grids during peaks in demand. While ensuring operational continuity of biotech operations, the latter would consequently reduce carbon footprint and enhance sustainability in general. Furthermore, the hybrid energy model delivers biotech data centers by providing scalability and flexibility where ability to rise with escalating power requirements does not place strain on the grid while keeping utilization of non-renewable energy resources at minimum.
Data Center Energy Efficiency Improvement
Energy consumption in biotech is mainly due to the inefficiency of data centers, especially their cooling systems. Those air-cooling methods, so traditional and long-employed until recent times, are insufficient for high-performance computing tasks in biotech. A more advanced approach utilizes hybrid cooling systems, merging liquid cooling with two-phase cooling.
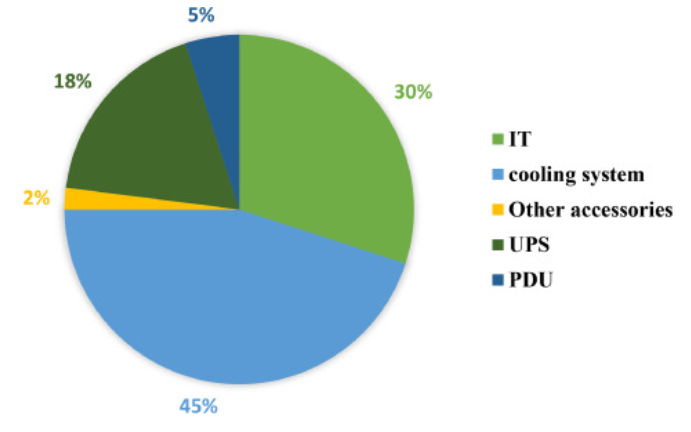
Liquid Cooling: Liquid cooling technology utilizes liquids that absorb heat more efficiently and transfer than air. It is of special importance in highly packed data centers wherein the traditional methods of cooling are not as efficient. Liquid cooling is superior in terms of heat transfer and thus minimizes the amount of energy needed to cool servers.
Two-phase Cooling: This process is based on evaporation that extracts heat from a cooling fluid and then condensation to recover the same fluid for re-use. Two-phase systems are quite effective in cooling high heat loads associated with AI computational processes, genomic sequencing and bioinformatics processes. This energy-dependent value of the cooling fluid is reduced thereby reducing overall energy.
Combining these cooling technologies will give biotech data centers a better energy efficiency, increased workload capacity, and the ability to scale without overloading their cooling infrastructure. These techniques can also be complemented with thermal energy storage systems, allowing for the storage of excess heat produced within peak hours to be used during off-peak hours, maximizing energy usage.
AI-Driven Workload Optimization
It has been used not only in biotech applications but also to handle and optimize energy use within the data centers. AI workload optimization utilizes advanced algorithms to predict server loads and better distribute computational tasks accordingly.
- Workload Forecasting: AI algorithms can predict future workloads using historical data and real-time inputs. Non-essential servers can be powered down during low-demand periods thereby saving energy, and for peak demand, dynamic resource allocation via AI can ensure energy efficiency in server operations. In this manner, data centers will not run at full capacity when it is not necessary, thus reducing a lot of energy.
- Cooling Prediction: In addition to the above, it can predict the amount of cooling required through an analysis of real-time temperature variations and server performance. The cooling system can then be dynamically adjusted according to the predicted cooling requirements in order to avoid energy wastage from unrequired cooling. Besides all the energy saved by way of consumption reduction, more years are added to the lifecycle of the cooling equipment as well.
- Load Balancing: AI balances workloads over the servers to not overload a single server. The chances of causing a power spike during such instances are minimal, which usually happens when such servers are pushed to their limits. Hence, more chances of more constant energy consumption and does not require cooling or more power increase due to AI-balanced workloads.
Cloud and Edge Hybrid Infrastructure
The other dominant solution to the management of biotech's energy consumption will be the adoption of a hybrid infrastructure model, which combines and offers the best features of both cloud computing and edge computing.
Cloud Computing: For high-scale bio-tech workloads, for instance, genomic sequencing and training of AI models, providers provide immense compute resources in scale across cloud providers such as AWS or Google Cloud to process the same vast quantities of data in a manner that is efficient, scalable, and fast. Also, the infrastructure can scale up or scale down as and when required, thus making optimum utilization of resources and at the same time minimizing energy wastage.
Local Edge Servers: Local Edge focuses on proximity to handle real-time data processing closer to the source of data. Local servers in the workflow of biotech could be used for preprocessing which might include filtering genomic data before it is sent out to the cloud for more analytical methods. This preprocessing will cut down the expense incurred by transferring data and the effort of energy expended when data is transferred over long distances. This would further facilitate security aspects of data, since the sensitive information may be processed on the local server, and only the agreed result is transferred out to the cloud.
This hybrid approach ensures that each platform is used efficiently in the tasks that it is optimally capable of performing; large, energy-intensive tasks are relegated to the cloud, while real-time local servers relate to the needs for data at hand, thus optimizing energy consumption across the biotech infrastructure.
Optimizing Quantum Computing for Biotech
Another front end for biotech is quantum computing, which has a capability no other machine has a capability to solve tasks in drug discovery and genomes analysis, among others. Quantum computing, however, is very energy-intensive especially extreme cooling requirements for quantum states.
- Renewable Power for Cryogenic Cooling: Quantum computers must be chilled almost down to absolute zero; therefore, biotech companies have to engineer efficient cryogenic cooling systems. Using renewable power sources like solar and wind power reduces the environmental degradation from quantum computing. This translates to quantum computers that deliver revolutionary results in biotech without contributing to the global energy crisis.
- Energy-Efficient Quantum Algorithms: Another area is that with energy usage in mind, another area to help reduce the energy consumption associated with quantum computing is the development of energy-efficient algorithms. These energy-efficient quantum algorithms are designed in a way where they need fewer resources to support their operations to minimize energy requirements. This is particularly crucial for AI-driven applications in drug discovery and bioinformatics, which may gain greater computational benefits while providing a greener footprint of energy use.
Mitigating Carbon Footprint: Cap-and-Trade Systems and Green Technology Investments
As biotech datacenter's grow, they must address their carbon emissions. One key strategy is engaging in Cap-and-Trade systems, where companies offset emissions by purchasing carbon credits from others that reduce theirs.
- Involuntary Markets: Regulated markets where companies must purchase credits if they exceed emissions limits. For example, biotech firms can buy credits from renewable energy producers to meet regulatory caps.
- Voluntary Markets: In these markets, companies voluntarily buy credits to improve their CSR profile, showcasing environmental responsibility and attracting eco-conscious investors.
Biotech companies can also reduce emissions by investing in green technologies like renewable energy and energy-efficient systems. This reduces reliance on offsets and directly lowers their carbon footprint. Benefits include:
- Environmental Stewardship: Demonstrating leadership in sustainability.
- Transparency: Blockchain tech ensures accountability in emissions trading.
- Supporting Innovation: Financing green projects accelerates renewable energy growth.
- Competitive Advantage: Sustainability attracts consumers and investors, enhancing profitability.
By combining cap-and-trade participation with green technology investments, biotech firms can significantly reduce their carbon footprint while maintaining growth and innovation.